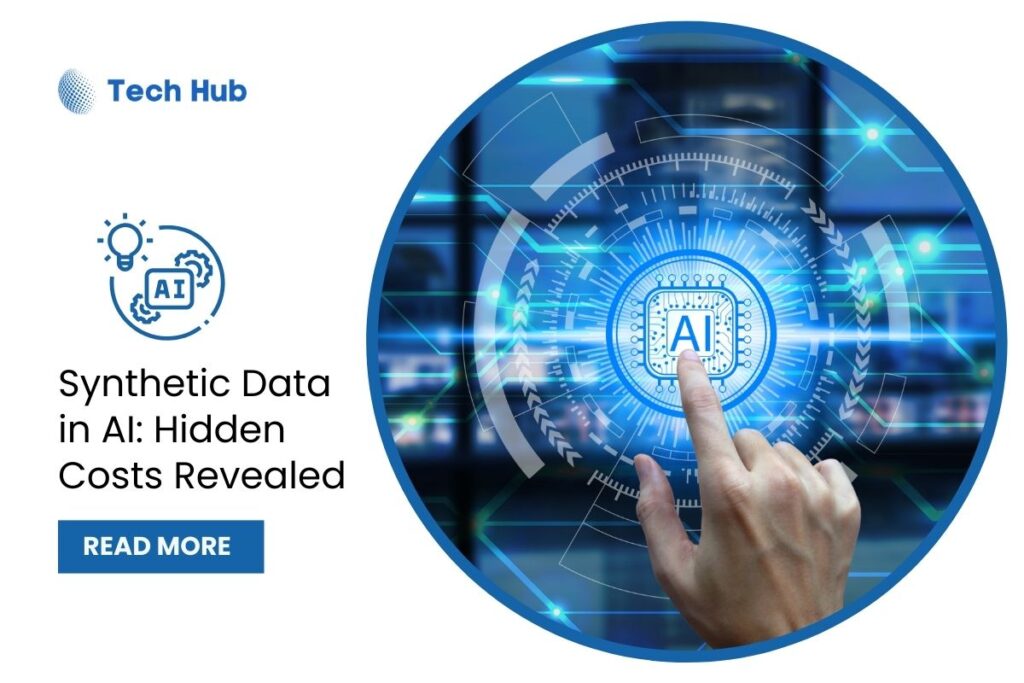
Last week, Elon Musk, the billionaire owner of X, claimed the supply of human-generated data for training artificial intelligence (AI) models like ChatGPT is running out. While Musk didn’t present evidence, similar concerns have been voiced by other tech leaders. Research suggests that human-generated data could be exhausted within two to eight years due to the rapidly growing demands of AI systems.
This shortage presents a significant challenge for AI development. With humans unable to produce data fast enough, the industry may increasingly rely on AI-generated “synthetic data.” While synthetic data offers potential solutions, it also poses risks to the reliability and accuracy of AI models if not managed carefully.
The Role of Real Data in AI Training
AI models rely on vast amounts of data—text, images, and videos created by humans—to improve their functionality. Real data is considered valuable because it captures real-world scenarios and contexts. However, it’s far from perfect.
Human-generated data can include inconsistencies, errors, and biases, which may lead AI models to produce inaccurate or skewed outputs. Moreover, preparing this data is labor-intensive. Collecting, labeling, cleaning, and organizing data can consume up to 80% of the development time for an AI system.
The increasing scarcity of real data, coupled with the surge in demand, has made it challenging for developers to keep up, forcing a shift towards synthetic alternatives.
Synthetic Data: Opportunities and Risks
Synthetic data, created by algorithms rather than humans, offers a fast and cost-effective solution for AI training. Unlike real data, synthetic data is virtually unlimited, and it can help address privacy and ethical concerns, especially for sensitive information such as health data.
However, synthetic data isn’t without its drawbacks. Overreliance on it can lead to “model collapse,” where AI systems produce inaccurate outputs or “hallucinations.” For instance, if an AI system generates flawed synthetic data, subsequent models trained on this data are likely to inherit and amplify those errors.
Another challenge is the lack of complexity in synthetic data. While real data reflects the diversity and nuances of real-world scenarios, synthetic data may oversimplify these details, reducing the utility of AI models trained on it.
Building Trustworthy AI with Synthetic Data
To harness the potential of synthetic data while mitigating its risks, international standards for data quality and validation are essential. Organizations such as the International Organization for Standardization (ISO) and the United Nations’ International Telecommunication Union (ITU) must establish robust frameworks to track and validate AI training data globally.
AI systems can also play a role in improving the quality of synthetic data. Algorithms could audit and compare synthetic data against real-world datasets to identify errors and ensure consistency. Additionally, human oversight is crucial during the training process to define objectives, validate data, and monitor model performance.
The Future of AI Relies on Data Quality
As the pool of human-generated data dwindles, synthetic data will inevitably play a larger role in training AI systems. If managed responsibly, synthetic data could enhance AI accuracy, reduce biases, and support innovation. However, its use requires careful oversight to maintain transparency, minimize errors, and uphold ethical standards.
The next era of AI depends on striking the right balance between real and synthetic data, ensuring these systems remain accurate, reliable, and trustworthy for users worldwide.